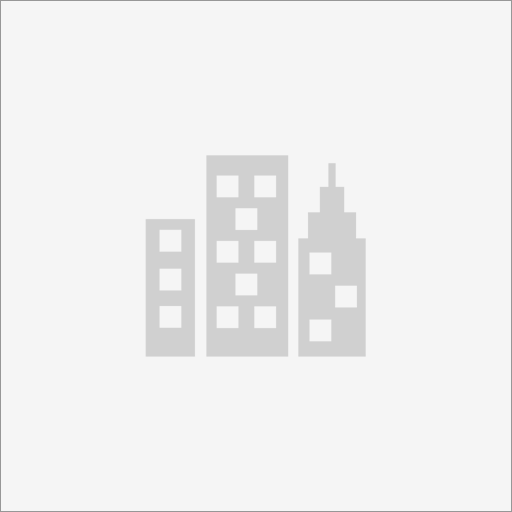
Institut Mines-Télécom
Job title:
Post-doctorant ou Post-doctorante en Génération de données de cyberattaques par interaction Humain-IA – CDD 2 ans
Company:
Institut Mines-Télécom
Job description
Post-doctorant ou Post-doctorante en Génération de données de cyberattaques par interaction Humain-IA – CDD 2 ansPostuler maintenantInstitut Mines-TélécomLieuil y a 18 jours Date de publicationil y a 18 joursS/O Niveau d’expérienceS/OType de contratCatégorie d’emploiABOUT TELECOM SUDPARISTelecom SudParis is a public graduate school for engineering, which has been recognized on the highest level in the domain of digital technology. The quality of its courses is founded on the scientific excellence of its faculty and on teaching techniques that emphasize project management, innovation and intercultural understanding. Telecom SudParis is part of the Institut Mines-Telecom, the number one group of engineering schools in France, under the supervision of the Minister for Industry. Telecom SudParis with Ecole Polytechnique, ENSTA Paris, ENSAE Paris and Telecom Paris are co-founders of the Institut Polytechnique de Paris, an institute of Science and Technology with an international vocation.Its assets include: a personalized course, varied opportunities, the no.3 incubator in France, an ICT research center, an international campus shared with Institut Mines-Telecom Business School and over 60 student societies and clubs. https://www.telecom-sudparis.eu/MISSIONSThe deployment of AI-based attack detection and classification facilitates the work of security analysts in Security Operation Centers (SOC) and Cyber Security Incident Response Teams (CSIRT) managing overwhelmingly large amounts of security reports, such as network activity logs. With AI-based engines, human analysts can identify and prioritize malicious activities quickly to keep up with the pace of attacks. Nevertheless, AI-based threat detection models still face several bottlenecks that need to be addressed, including the insufficient coverage of attack behaviours. AI models require large amounts of training data to cover as many attack behaviours as possible. However, in the practice of cybersecurity, the deployed probes cannot guarantee to provide comprehensive coverage over different attack behaviours, especially emerging new attacks. The lack of coverage over different attack behaviours, i.e. the issue of out-of-distribution samples in attack detection, makes it challenging to accurately categorise the threats that are being faced.In the framework of the CKRISP project (ANR TSIA call), we will leverage the attack knowledge and the causal relationships between security incidents from a cybersecurity knowledge graph (CSKG) to create an AI-driven attack data generation method. Our approach involves developing a reinforcement learning-based attack behaviour prediction policy model. This model will be capable of mimicking cyberattack strategies used by human attackers/analysts (for penetration tests). Additionally, the model should also explore new attack methods based on the contextual knowledge of the target assets. By synthesizing attack data using this approach, we can help human experts explore possible attack paths that may not appear in previous observations. We will not only enhance the detection coverage of AI-based IDS but also enable human analysts to identify potential vulnerabilities.CKRISP will establish a human-AI cooperative process to address the bottleneck of attack behaviour exploration and prediction. First, new attacks beyond human analysts’ awareness, e.g., zero-day attacks, may exist in the observed behaviour logs. Second, most of the security events collected in practice can be unlabelled and/or incomplete due to the imperfection of probes. Inspired by the recent success of LLM-based AI applications, the expected human-AI cooperative will achieve:1) the exploration of subgraph structures of CSKGs to unveil possible attack paths;2) the promotion of human verification of suspicious behaviours unveiled by AI systems and the concatenation of human analysts’ knowledge to guide attack exploration with active learning and3) the recovery of missing entities in CSKG by adopting LLMs to estimate the missed attack data or to produce synthetic attack behaviours.ACTIVITIESThe proposed work focuses on generating attack data by combining the CSKG produced in CKRISP and human analysts’ knowledge from two perspectives. First, we will use pre-trained LLMs with the help of CSKG to produce attack data. Second, we will develop a language mapping between the actions supported by the reinforcement learning agent trained to explore the knowledge graphs and a penetration testing framework to generate attack payloads.The contribution attempts at building an AI agent to query an attack/malware knowledge graph, in order to achieve attack behaviour prediction/categorisation. The agent may be trained using Reinforcement Learning or combined with an LLM for querying the attack knowledge graph. A GNN model can also be considered to generate soft prompt input to a LLM to achieve understanding/querying an attack knowledge graph. Predicting/categorising the attack behaviour can reach the generation of attack behaviour data, e.g. generation of port scans or DDoS attack network traffic flows using the attack knowledge graph.In summary, the work will use the attack knowledge graph built in CKRISP as a knowledge base, and use the AI agent-to-build to summarize the attack behavioural patterns. These patterns will finally be used for a two-fold goal: predict attack behaviours and generate attack data.LEVEL OF TRAINING REQUIRED
- PhD for less than 3 years
REQUIRED SKILLS AND KNOWLEDGE
- Experience in machine-learning based cybersecurity, in particular, intrusion detection
- Knowledge in large language models, graph neural networks or reinforcement learning
- Knowledge in knowledge graphs
- English written and spoken
ADVANTAGEOUS SKILLS, KNOWLEDGE AND EXPERIENCE:
- Prior experience with testbed or data generation
SKILLS AND ABILITIES
- Rigor
- Autonomy
- Teamwork
APPLICATION PROCEDURE
- Application deadline: September 30st, 2024
- Localisation: PALAISEAU
- Nature of the contract: 24-month renewable fixed-term contract
- Category and profession of the position: II – P, Post-doctoral
- To apply, please send us a CV, a cover letter and a summary of your doctoral thesis
- The positions offered for recruitment are open to all with, on request, accommodations for candidates with disabilities
Balises associées–RÉSUMÉ DE L’ OFFREPost-doctorant ou Post-doctorante en Génération de données de cyberattaques par interaction Humain-IA – CDD 2 ansInstitut Mines-TélécomPalaiseauil y a 18 joursS/OTemps pleinPostuler maintenantEnregistrerSignaler cet emploiDemandeurs d’emploiEmployeursInformations légalesEntrepriseRechercher(modifier les préférences)© Copyright 2024 LesJeudis. Tous droits réservés. Toutes les autres marques déposées (logos, etc.) d’entreprise appartiennent à leurs propriétaires et ayants droits respectifs.
Expected salary
Location
Palaiseau, Essonne
Job date
Tue, 03 Sep 2024 07:29:29 GMT
To help us track our recruitment effort, please indicate in your email/cover letter where (vacanciesineu.com) you saw this job posting.